Order the steps required to predict the volume – Predicting volume is a crucial aspect of business planning and forecasting. By following a structured approach, businesses can enhance the accuracy of their volume predictions and make informed decisions. This comprehensive guide Artikels the essential steps involved in order to predict volume effectively, providing a roadmap for businesses to navigate the complexities of demand forecasting.
The subsequent paragraphs delve into each step, exploring the techniques and methodologies employed to gather data, analyze trends, identify causal factors, develop forecasting models, evaluate their accuracy, and refine them over time. Additionally, data visualization plays a pivotal role in presenting insights and evaluating model performance, ensuring that businesses can make data-driven decisions with confidence.
Predicting Volume: A Comprehensive Guide: Order The Steps Required To Predict The Volume
Predicting volume is a critical task for businesses to optimize inventory management, supply chain operations, and marketing strategies. This guide provides a comprehensive overview of the steps involved in predicting volume, from data collection to model evaluation and refinement.
Data Collection
Gathering accurate and reliable data is the foundation for effective volume prediction. This includes historical sales data, market trends, and relevant industry data. Data cleaning and organization are crucial to ensure data integrity and consistency.
Time Series Analysis
Time series analysis involves statistical techniques to analyze historical sales patterns. Moving averages, exponential smoothing, and ARIMA models are commonly used to identify trends, seasonality, and other patterns in sales data.
Causal Analysis
Identifying factors that influence sales volume, such as economic indicators, marketing campaigns, and competitor activity, is essential. Regression analysis and other statistical techniques can quantify the impact of these factors on sales.
Forecasting Models
Various forecasting models are available, including linear regression, time series models, and machine learning algorithms. The choice of model depends on the characteristics of the data and the desired level of accuracy.
Model Evaluation and Refinement, Order the steps required to predict the volume
Evaluating the accuracy of forecasting models using metrics such as mean absolute error and root mean squared error is crucial. Refinement techniques, such as parameter tuning and ensemble methods, can improve model performance over time.
Data Visualization
Visualizing key insights and trends from data analysis enhances understanding and communication. Charts and graphs can illustrate forecasting model results and evaluate their accuracy.
Answers to Common Questions
What are the key steps involved in predicting volume?
The key steps include data collection, time series analysis, causal analysis, forecasting model selection, model evaluation, and refinement.
How can businesses improve the accuracy of their volume predictions?
By following a structured approach, utilizing appropriate statistical techniques, and considering relevant factors that influence volume.
What role does data visualization play in volume prediction?
Data visualization helps businesses present key insights, trends, and model performance, enabling them to make informed decisions based on visual representations of data.
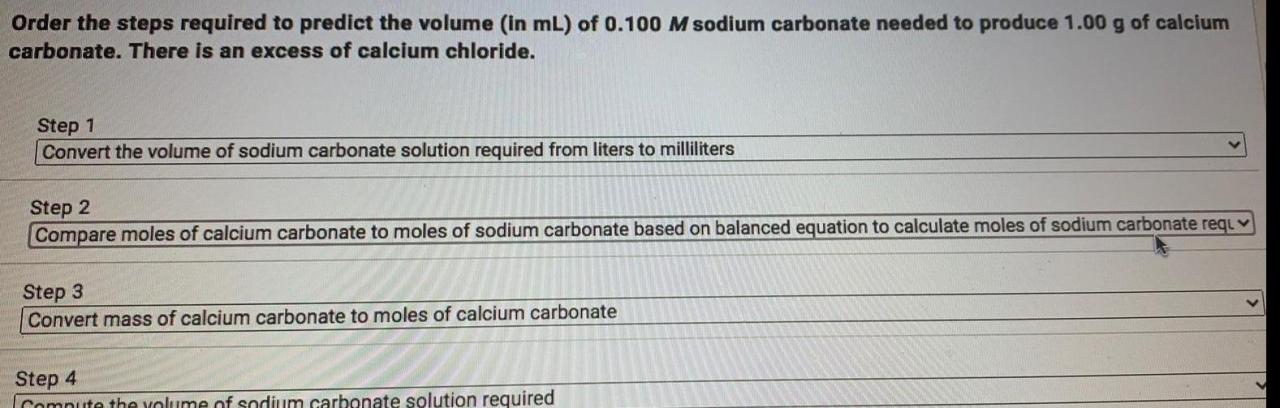
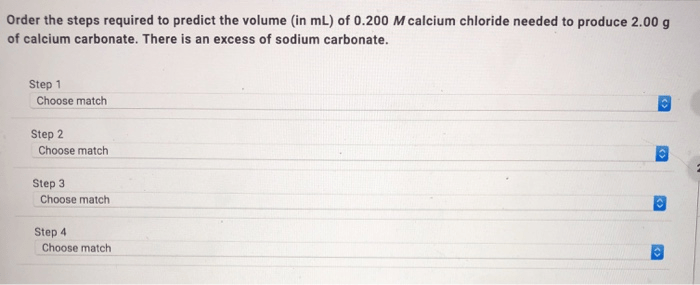
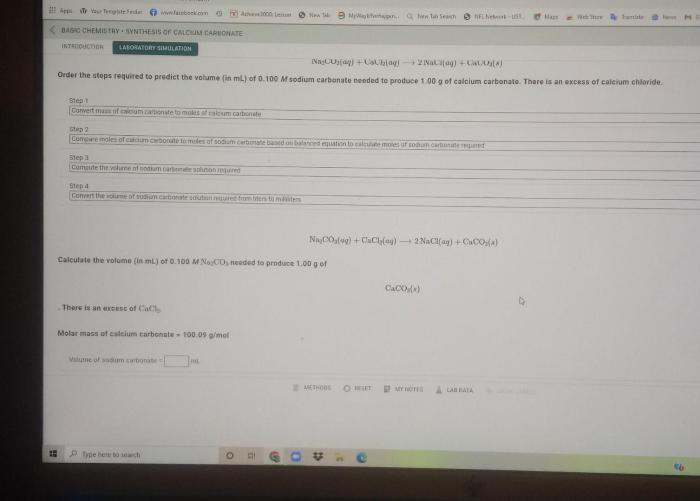